In the dynamic landscape of AI evolution, transparency in AI stands out as a cornerstone for building ethically aligned systems. Embracing the dimensions of openness is critical to ensure that AI models and algorithms operate with clarity and are understandable to their human counterparts.
A Brief for the Conscientious AI Explorer:
- Transparency in AI is not merely about open algorithms but about creating systems where decisions can be understood, questioned, and validated. Such clarity is essential to confront potential biases, reinforce trust, and advocate for responsible AI applications across myriad domains.
- For AI enthusiasts and professionals alike, understanding these dimensions is a requisite. A thorough insight into transparency not only bolsters public trust but also guides the trajectory of AI towards more democratic and inclusive innovations.
By actively promoting and adhering to transparency principles in AI, entities anchor themselves as torchbearers of ethical AI practices. This commitment ensures that as AI continues its onward march, it does so with the weight of accountability, fostering a digital ecosystem where ethics and progress go hand in hand.
Welcome to the second article in the Ethics and AI Series. These Blue Lane Group posts aim to shed light on the multifaceted dimensions of AI ethics, guiding professionals and enthusiasts in navigating this intricate landscape with informed decisions and practices.

Disclosure: The digital products mentioned in this article are highly regarded in the marketplace and are endorsed by the Blue Lane Group staff. We may earn a commission at no additional cost if you purchase through the provided links.
Table of Contents

Introduction: Grasping the Significance of Transparency in AI
Artificial intelligence stands as a cornerstone in the ongoing technological renaissance. As it progressively integrates into healthcare, finance, education, and countless other sectors, the call for clarity in its operations intensifies. While its capabilities are awe-inspiring, the enigmatic nature of AI can sometimes lead to apprehensions.
Products like the Google What-If Tool and IBM AI Explainability 360 have emerged as the industry’s answer to these concerns. They represent a burgeoning movement towards making AI transparent, decipherable, and trustworthy. With such tools, users can delve into the intricate mechanisms of AI, fostering trust and ensuring ethical alignment.
As the tendrils of AI touch every facet of human existence, the onus of ensuring transparency falls on developers, regulators, and educators alike. For AI to truly integrate into society’s fabric, it must be understandable, predictable, and transparent.

What does transparency in AI entail, and why is it vital?
Transparency in AI goes beyond just opening the hood and revealing the gears. It ensures that users, stakeholders, and even laypeople can understand, predict, and trust AI systems. This comprehensibility extends from the algorithm’s design, the data it’s trained on, to the predictions it makes.
DARPA’s XAI (Explainable AI) stands as a testament to the importance of this principle. This program strives to cultivate AI systems that are not only high-performing but also inherently understandable. With such transparency, users are better positioned to trust the system, leading to more effective human-AI collaborations.
The implications of a non-transparent AI can be vast, ranging from unintentional biases in decision-making to unwarranted trust in inaccurate predictions. Hence, ensuring transparency is not just an ethical imperative but a foundational necessity for AI’s widespread adoption and success.
The intertwined journey of AI advancements and transparency needs
The history of AI is a tapestry of remarkable innovations, breakthroughs, and, equally, challenges. As AI’s capabilities burgeoned, so did the complexities of its inner workings. Tools like BERTViz and DeepLIFT became essential, enabling researchers and developers to visualize and understand these complexities.
Yet, the demand for clarity and transparency escalated as the models grew more intricate. The industry’s multifaceted response involves tools, guidelines, regulations, and training programs. The Fairness Indicators, for instance, help teams ensure model fairness, aligning AI predictions with ethical and transparent benchmarks.
This journey underscores a vital lesson: as AI evolves, its transparency needs will shift, demanding perpetual vigilance, innovation, and adaptability from all involved stakeholders.

The 12 Ethical Dimensions of Transparency in AI
Transparency in artificial intelligence is a multifaceted topic beyond just making algorithms understandable. It encompasses various ethical dimensions that address the concerns and aspirations of developers, users, and the broader society.
Understanding these dimensions becomes paramount as AI systems grow in complexity and influence. Let’s explore the core ethical aspects that define transparency in AI and explore the essence of each dimension.
1. Algorithmic Understandability: Decoding AI Processes
In the dynamic world of artificial intelligence, ensuring that algorithms are comprehensible remains a pivotal challenge. Algorithmic understandability refers to the clarity humans can interpret and grasp AI processes.
As AI continues to permeate diverse sectors, from healthcare to finance, the onus lies on developers to build easily decoded models. Tools like DeepLIFT and BERTViz offer glimpses into the intricate decision-making processes of AI, aiding researchers in unraveling the layers of computations. However, the pursuit doesn’t end with creating transparent algorithms.
The challenge also entails ensuring that individuals can derive insights from these models irrespective of their technical prowess. By prioritizing algorithmic understandability, we foster trust and empower users to leverage AI, understanding its recommendations and potential limitations confidently.
2. Accountability: Assigning Responsibility in AI Systems
In AI, accountability resonates with the fundamental question: Who takes responsibility when things go awry? Transparency in AI isn’t merely about showcasing how algorithms function but also delineating clear lines of responsibility.
Whether it’s an AI model inadvertently promoting bias or a system failure leading to operational hiccups, having a robust accountability framework ensures that errors can be rectified promptly. Furthermore, programs like DARPA’s XAI emphasize making AI more interpretable and ensuring users can trust and rely on its decisions.
As AI systems integrate deeper into our daily lives, precise mechanisms to address grievances, correct mistakes, and continually improve become indispensable. When intertwined with transparency, accountability paves the way for AI systems that are efficient and ethically sound.

3. Bias Mitigation: How Transparency Aids Fair AI Outcomes
Both latent and overt bias can seep into AI systems, leading to skewed and often unfair outcomes. Transparency plays an instrumental role in identifying and addressing these biases. By clarifying the workings of AI systems, developers and users can spot potential prejudices in how the AI evaluates and responds.
Tools such as Fairness Indicators aim to improve model fairness by offering consistent and transparent predictions across diverse user groups. But bias mitigation isn’t a one-time activity. It requires continuous efforts to assess, refine, and retrain AI models to ensure equitable results.
Emphasizing transparency in AI not only aids in pinpointing biases but also provides avenues to rectify them, leading to fairer and more just AI outcomes for all.
4. User Trust: Establishing Confidence through Transparent AI
The adoption and success of AI largely hinge on the trust users place in these systems. A transparent AI serves as the foundation for building and nurturing this trust. When users can understand how an AI system operates and arrives at its decisions, their confidence in its capabilities naturally amplifies.
Visualization tools like the Google What-If Tool facilitate understanding by allowing users to interact with and probe machine learning models. Moreover, trust isn’t just a byproduct of transparency; it’s a continuous relationship cultivated through consistent, clear, and reliable AI operations.
Ensuring that AI systems function predictably, ethically, and transparently fosters an environment where users feel safe, informed, and confident in integrating AI into their tasks and decision-making processes.
5. Decision Justification: How AI Arrives at its Conclusions
One of the pressing concerns in AI adoption is understanding the rationale behind the decisions AI systems make. Tools like SHAP (SHapley Additive exPlanations) elucidate the output of machine learning models by assigning importance values to each feature, helping in demystifying the AI’s conclusions.
Decision justification goes beyond mere transparency. It provides stakeholders with insights into why certain decisions are favored over others. As AI systems increasingly influence medical diagnoses and financial lending, the ability to justify decisions becomes paramount. This builds confidence among end-users and ensures that AI operates within an acceptable ethical framework.

6. Data Sourcing: Ensuring Ethical Gathering and Use of Information
Data is the foundational block on which algorithms are trained and fine-tuned at the heart of every AI system. Ethical and transparent data sourcing is crucial to the integrity and reliability of AI outcomes. The tools and frameworks to gather data must adhere to established ethical guidelines, ensuring the information is sourced responsibly and without biases.
However, it’s not just about gathering data ethically; how it’s utilized within AI systems also matters. Transparent practices in data handling ensure that personal and sensitive information remains protected while enabling AI to learn and adapt. Prioritizing ethical data sourcing and usage safeguards user trust and upholds the moral standards of AI applications.
7. Model Training: Opening the Black Box of AI Learning
The phrase ‘black box’ is often associated with AI systems, hinting at their opaque nature. But strides in AI transparency aim to open this box, illuminating the intricate processes involved in model training. Tools like InterpretML and TensorFlow Model Analysis are pivotal in making AI training more comprehensible.
Understanding how AI models learn and evolve is crucial for developers and end-users. It ensures the system’s learning aligns with desired outcomes and ethical standards. More importantly, transparent model training enables continuous refinement, ensuring that AI systems remain relevant, efficient, and ethically sound as they adapt to new data and challenges.
8. Regulatory Compliance: Meeting Standards with Transparent Practices
With the rapid evolution of AI, regulatory bodies worldwide emphasize the need for standardized, transparent practices. Compliance isn’t just about adhering to rules; it’s about ensuring that AI systems are developed and operated within a framework that prioritizes user safety, fairness, and ethics.
Transparent AI practices simplify the path to regulatory compliance. By maintaining clear documentation and ensuring open communication about how AI systems function, developers can more easily navigate the intricate landscape of regulations. Emphasizing transparent practices not only minimizes legal risks but also reinforces the commitment to creating AI systems that are beneficial, fair, and trustworthy for all users.

9. Privacy Protection: Balancing AI Clarity with Data Security
The balance between AI transparency and data privacy is a delicate dance. While users and stakeholders need to understand how AI systems operate, this clarity should never come at the expense of compromising personal data. Tools such as Google What-If Tool promote AI transparency without revealing sensitive information.
As AI systems delve deeper into user data to make accurate predictions and provide personalized experiences, safeguarding user privacy becomes paramount. This involves implementing robust encryption methods, ensuring data anonymization, and adhering to global data protection regulations. Striking the right balance is essential to uphold user trust and pave the way for AI’s ethical progression.
10. Stakeholder Communication: Engaging Parties in AI Development
Transparent AI is about technology, open dialogue, and collaboration. Stakeholder communication plays a pivotal role in ensuring AI’s responsible growth. Platforms such as DARPA’s XAI (Explainable AI) foster understanding, ensuring stakeholders remain informed and involved in AI’s trajectory.
Whether it’s developers, end-users, or regulatory bodies, every party has a stake in AI’s future. Transparent communication ensures everyone is on the same page, fostering an ethical, fair, and beneficial collective vision for AI. Through continuous engagement, the AI community can collaboratively address challenges, innovate, and steer AI towards a future that benefits all.
11. Evolution and Adaptability: AI’s Ongoing Transparent Journey
AI’s landscape is ever-evolving, characterized by continuous advancements and shifts. Ensuring transparency isn’t a one-time task but an ongoing journey. Tools such as Manifold and Netron contribute to this journey by offering visual insights into AI’s learning processes, allowing adaptability.
Maintaining transparency becomes even more crucial as AI systems grow and adapt to new data, challenges, and environments. It allows for timely refinements, ensuring AI’s evolution aligns with ethical standards and societal needs. The commitment to transparency ensures that as AI advances, it remains understandable, trustworthy, and aligned with human values.
12. Global Perspectives: Addressing Transparency Needs Across Borders
AI’s impact isn’t limited to a specific region or country; it’s a global phenomenon. Addressing transparency, therefore, requires a perspective that transcends borders. Tools like IBM AI Explainability 360 cater to diverse user groups, ensuring that transparency standards are consistent, irrespective of geography.
As AI systems cater to global audiences, understanding and addressing cultural, ethical, and regulatory nuances become crucial. By fostering a global perspective on transparency, the AI community can ensure that systems are technically sound, culturally sensitive, and universally accessible. Embracing an international outlook is the key to developing AI systems that are universally ethical, transparent, and beneficial.

Strategies to Enhance Transparency in AI Systems
In the ever-evolving realm of artificial intelligence, transparency remains a critical cornerstone. As AI systems permeate every facet of our lives, they must operate transparently, fostering trust and ensuring ethical operations.
To achieve this transparency, various strategies come into play, encompassing everything from design principles to stakeholder education. The following sections delve deep into these strategies, shedding light on the roadmap to a transparent AI ecosystem.
Implementing Transparent Design Principles in AI
The foundation of any transparent AI system lies in its design principles. Leveraging tools such as InterpretML and SHAP, developers can incorporate transparency right from the onset. These tools offer invaluable insights into AI decision-making, ensuring that the underlying processes are understandable and open to scrutiny.
Transparent design involves selecting suitable algorithms and ensuring that the entire AI pipeline, from data collection to model deployment, upholds transparency standards. By adhering to evident design principles, AI systems can ensure ethical operations, foster user trust, and meet regulatory requirements. A proactive approach sets the stage for AI’s responsible and transparent growth.

Educating developers and stakeholders on transparency
Understanding the importance of transparency is half the battle; the other half involves disseminating this understanding. Through initiatives like DARPA’s XAI (Explainable AI), developers and stakeholders can be educated on the nuances of transparent AI operations.
Education isn’t just about tools and techniques; it’s also about fostering a culture of transparency. By ensuring developers and stakeholders understand the ethical, regulatory, and practical implications of transparency, they can collaboratively work towards AI systems that are not only effective but also ethically sound. Informed stakeholders translate to AI systems that prioritize transparency at every turn.
Prioritizing clear, user-friendly AI interfaces
For the end-user, transparency often manifests through AI interfaces. Using visualization tools like BERTViz and the Google What-If Tool, developers can create user-friendly interfaces that offer a clear window into AI decision-making processes. These interfaces demystify AI operations, making them accessible to users from all walks of life.
A straightforward, intuitive AI interface ensures that users can understand and trust the AI systems they interact with. By prioritizing user-friendliness, developers can ensure that transparency isn’t just a backend feature but a front-and-center user experience. It’s about making transparency tangible, accessible, and user-centric.
Building diverse and inclusive AI development teams
Transparency in AI is as much about diverse perspectives as algorithms and tools. By fostering varied and inclusive AI development teams, organizations can ensure that their AI systems are free from biases and cater to a broad user base. Tools like Fairness Indicators can assist, but human diversity remains paramount.
A diverse team brings varied perspectives, ensuring that AI systems are developed with a broad user base in mind. This inclusivity promotes transparency and ensures that AI systems are ethical, fair, and representative. Embracing diversity is a strategic move, ensuring that transparency in AI benefits all, devoid of biases.

Essential FAQs: Addressing Concerns on Transparency in AI
In the intricate world of artificial intelligence, transparency has become a subject of great importance and equally great debate. As AI’s prominence grows, so do the questions surrounding its ethical and transparent functioning.
This section addresses some of the most pressing concerns and frequently asked questions about transparency in AI, providing clarity and insights for both AI enthusiasts and skeptics alike.
1. What challenges impede achieving full transparency in AI?
Achieving full transparency in AI is a multifaceted challenge. One of the primary issues is the inherent complexity of advanced AI models and profound learning algorithms. Their multi-layered structures make it challenging to decipher how decisions are made.
Additionally, there’s a trade-off between performance and transparency: simpler models are often more interpretable but may not perform as well as complex ones. Commercial interests also play a role, as businesses might be reluctant to disclose proprietary information about their AI systems.
Lastly, even when transparency tools are available, there may be a lack of expertise or resources to implement them effectively in various organizations.
2. How does transparency in AI impact user trust?
Transparency is intrinsically linked to user trust. When users, whether consumers or businesses understand how an AI system makes decisions, they are more likely to trust its outputs. Transparent AI offers accountability, allowing users to ascertain whether the AI functions as intended and if its decisions are based on logical and unbiased factors.
In contrast, an opaque AI system, where the decision-making process is hidden, can lead to skepticism and reluctance to adopt. Furthermore, in cases where AI errors occur, transparency can pave the way for quicker resolutions and reaffirm user trust.

3. Are there established standards for AI transparency?
Yes, there are emerging standards for AI transparency, although they are still in developmental stages. Organizations like the IEEE and OpenAI have proposed guidelines and principles to ensure the ethical and transparent development of AI systems.
Specific sectors, especially those heavily regulated, like finance and healthcare, have more stringent guidelines to ensure AI transparency and accountability. It’s also worth noting that as the AI field evolves, so will the standards, emphasizing adaptability to accommodate new challenges and technologies.
4. How do businesses balance transparency with proprietary algorithms?
Balancing transparency with proprietary interests is a delicate act for businesses. On one hand, businesses want to ensure user trust by being transparent about how their AI systems operate. On the other, they need to protect their intellectual property, which might give them a competitive edge. One approach is to provide high-level insights into how the AI system functions without revealing the intricacies of the algorithm.
Open-source models offer another avenue where the core algorithm is public, but specific implementations or datasets remain proprietary. Additionally, third-party audits can be conducted to verify the transparency and ethics of an AI system without revealing its proprietary details.

Conclusion: Fostering an Ethical Future with Transparent AI
In the realm of technological advancements, artificial intelligence stands out as one of the most transformative and, at times, controversial innovations. As AI systems increasingly permeate our daily lives, from personalized recommendations to complex decision-making processes, the call for transparency becomes louder and more urgent.
Transparency in AI is not just about understanding algorithms or deciphering code. It’s about cultivating trust, ensuring fairness, and promoting responsible innovation. As we have seen, transparency touches on many ethical dimensions, ranging from accountability to bias mitigation, data sourcing, and global perspectives.
Each dimension underscores the importance of clarity and openness in AI development, ensuring that these systems align with human values and societal norms.
However, achieving full transparency in AI is not without its challenges. From the complexity of deep learning models to the commercial interests of businesses, various factors can impede the path to full disclosure. Yet, these challenges are not insurmountable. We can foster an environment where AI systems are high-performing and transparent through collective effort, collaboration, and a commitment to ethical principles.
Ultimately, the journey towards transparent AI is a testament to our commitment to an ethical future. A future where AI augments human capabilities and resonates with our shared values of fairness, inclusivity, and trust.
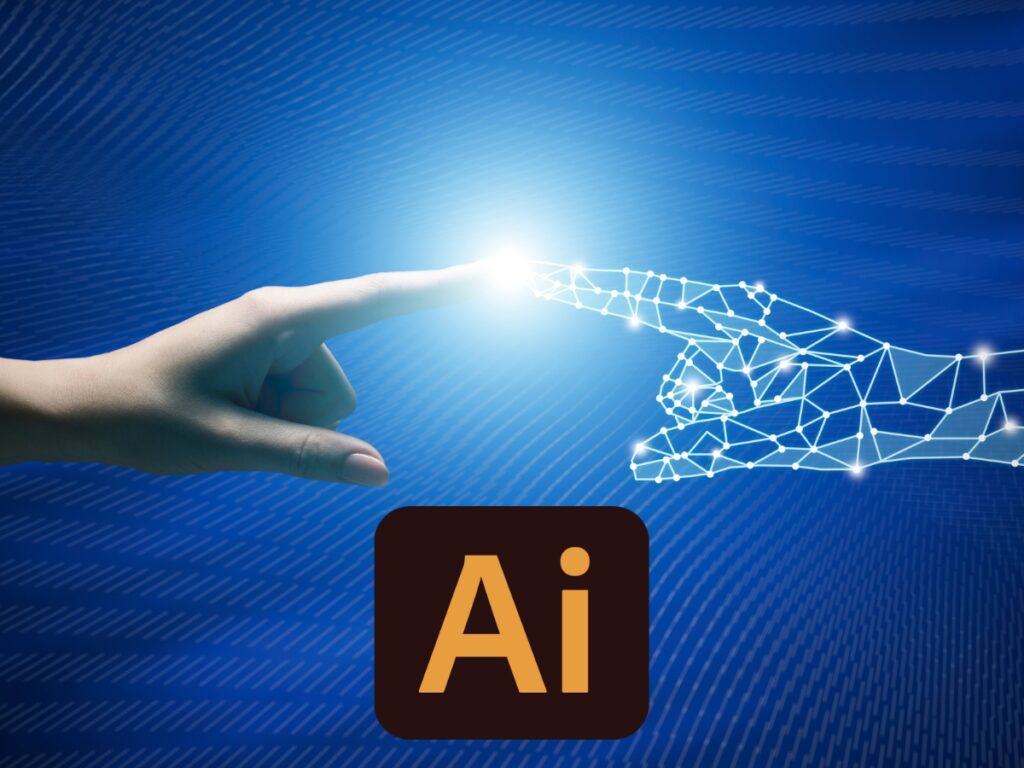
You Might Also Like:
If you enjoyed this article and received value from it, check out the other Blue Lane Group articles in the Ethics and AI Series:
- Unraveling 10 Core Ethical Concerns in AI Every Expert Must Know
- Shedding Light on the 10 Major Ethical Dilemmas of Data Privacy in AI
- Unlocking 12 Ethical Dimensions Behind Transparency in AI
- Dive Deep: 10 Ethical Dilemmas in Modern AI Development Tools
- Master 11 Essential Principles for Ethical Fairness in AI
- Deciphering the Ethical Maze: 9 Aspects of AI Data Annotation
- Navigate the 9 Ethical Dilemmas of AI and Modern Reputation Scores
- Mastering the 11 Nuances of AI Ethics in Healthcare Practices
- Decoding 11 Key Insights into AI Ethical Guidelines for Businesses
- Unveiling the Future: 10 AI Trends Shaping Ethics in NLP
- Revealing the 9 Ethical Challenges of Security Surveillance in AI Today
- Navigating the Future: 10 Trends Shaping Ethics in Fair AI Systems
- Navigating the Future: 12 Trends Driving AI Employment Ethics
- Exploring 10 Pivotal Issues of AI in Advertising Ethics Today
- Illuminate: 9 Innovative Trends Shaping Ethics in Intellectual Property Rights
- Navigating the Future: 10 Trends Shaping Ethics in AI in Crime Prevention
- Dissecting the 11 Key Ethical Boundaries in Deepfakes and AI
- Unraveling 10 Ethical Implications of AI and Vehicles Today
- Decoding 9 Critical Ethical Practices in Building Trust in AI Systems
- Highlighting 11 Pivotal Ethical Implications in AI Globalization
- Unveiling 10 Ethical Foundations in AI Research Methods Today